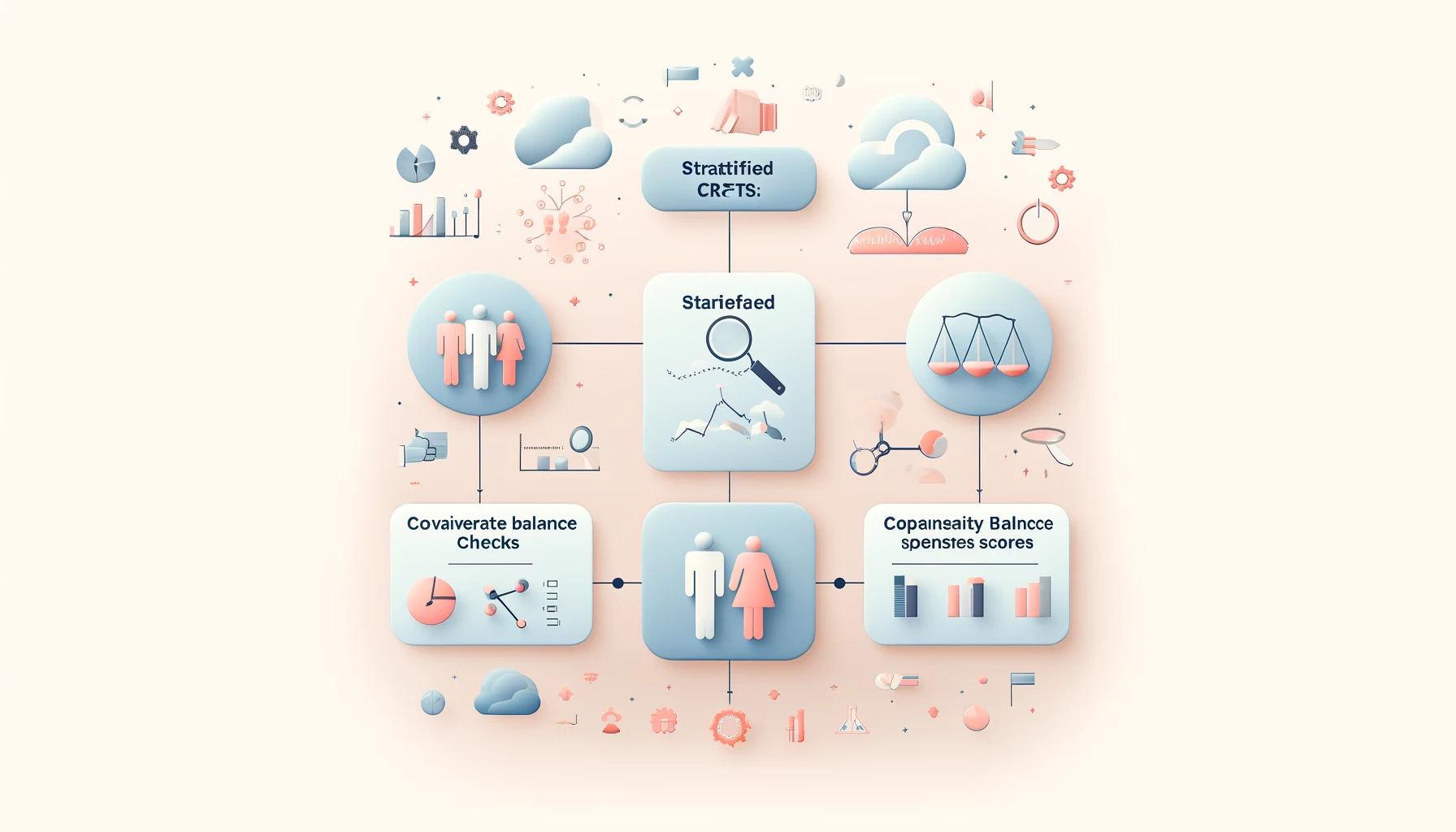
Causal ML Book: Summary of Key Concepts from Chapter 5
Causal inference is a cornerstone of research and analysis in many fields, from economics to medicine to social sciences. Chapter 5 of the Causal ML Book delves into advanced methodologies that ensure comparability between treatment and control groups, which is crucial for valid causal inference. This post will summarize the key concepts discussed in Chapter 5, focusing on Stratified Randomized Controlled Trials (RCTs), Covariate Balance Checks, Conditioning on Propensity Scores, and the importance of the overlap assumption.
Introduction to Conditional Ignorability
Conditional ignorability is a foundational concept in causal inference, enabling the estimation of causal effects from observational data by controlling for certain variables (covariates). This concept allows researchers to mimic the conditions of a randomized controlled trial (RCT) within observational studies, ensuring that the treatment assignment is as good as random once covariates are accounted for.
Causal Inference and Conditional Ignorability:
- Causal Inference: Determining whether a relationship between two variables is causal rather than merely correlational.
- Conditional Ignorability: Assuming that, after controlling for certain covariates, the treatment assignment is as good as random.
This approach is particularly important in scenarios where randomized controlled trials are not feasible, such as studying the effects of educational programs or medical treatments in real-world settings.
Potential Outcomes and Ignorability
The potential outcomes framework, introduced by Donald Rubin, is essential for defining and estimating causal effects. It considers the outcomes that would occur under different treatment conditions. Ignorability (or unconfoundedness) assumes that, given covariates XX, the treatment assignment is independent of the potential outcomes. This assumption allows researchers to estimate causal effects by comparing treated and untreated individuals with similar covariates, thereby controlling for confounding bias.
Example:
- Potential Outcomes with Training: Y(1) is the income an individual would earn if they participated in a job training program.
- Potential Outcomes without Training: Y(0) is the income the individual would earn if they did not participate in the training program.
- Causal Effect: The difference δ=Y(1)−Y(0)
Identification by Conditioning
Identification by conditioning involves estimating causal effects in observational studies by controlling for confounding variables. By stratifying the data based on covariates X, researchers can compare outcomes within these strata to isolate the effect of the treatment. This method relies on the ignorability assumption, which posits that treatment assignment is as good as random within each level of X.
Steps for Identification by Conditioning:
- Identify Covariates X: Determine the set of covariates that influence both the treatment and the outcome.
- Stratify by X: Divide the data into groups based on the values of X.
- Compare Outcomes within Strata: Compare the outcomes of treated and untreated individuals within each group.
- Aggregate Effects: Combine the within-group estimates to get an overall estimate of the causal effect.
Conditional Ignorability via Causal Diagrams
Causal diagrams, or Directed Acyclic Graphs (DAGs), visually represent the assumptions and relationships among variables in a causal inference study. These diagrams help identify confounders and illustrate how conditioning on certain variables can remove bias. By blocking all backdoor paths in the diagram, researchers can ensure that the treatment is independent of the potential outcomes given the covariates.
Example Diagram:
- Variables: Job training program (D), income (Y), prior job performance (X), and an unmeasured variable (U).
- Diagram:
- U → D → Y
- U → X → Y
- X → D
- Blocking Paths: Conditioning on X blocks the backdoor paths from D to Y, ensuring D is independent of Y given X.
Connections to Linear Regression
Linear regression techniques can be used to estimate causal effects when the ignorability assumption holds. By modeling the relationship between the outcome Y and the treatment D, along with covariates X, researchers can estimate the average treatment effect. This approach bridges traditional linear regression models with modern causal inference methods.
Practical Steps:
- Fit the Regression Model: Estimate the coefficients by fitting the linear regression model to the data.
- Interpret the Coefficients: The coefficient representing the treatment effect is the average treatment effect if the ignorability assumption holds.
Example:
- Model: Y=αD+β1(Prior Performance)+β2(Education)+β3(Age)+ϵ
- Estimate: Fit the model to estimate α, β1, β2, and β3.
- Interpret: The estimate of αα is the average treatment effect of the training program on income.
Identification Using Propensity Scores
Propensity scores provide an alternative method for estimating causal effects when the treatment assignment is not random. The propensity score p(X) is the probability of receiving the treatment given the covariates. By using methods such as matching, stratification, and weighting, researchers can balance the covariates between treated and untreated groups, facilitating unbiased causal inference.
Methods Using Propensity Scores:
- Matching: Pair treated and untreated individuals with similar propensity scores.
- Stratification: Divide the sample into strata based on propensity scores and estimate the treatment effect within each stratum.
- Weighting: Use inverse probability weighting to create a balanced sample and estimate the treatment effect.
Example:
- Estimate the Propensity Score: Use logistic regression to estimate the probability of receiving the training program.
- Apply a Method: Match, stratify, or weight individuals based on their propensity scores to balance covariates between groups.
Overlap Assumption
The overlap (or common support) assumption ensures that there are both treated and untreated individuals for every combination of covariates, allowing for meaningful comparisons. Without overlap, it is impossible to estimate causal effects in regions of the covariate space where only treated or only untreated individuals exist.
Example:
- With Overlap: Individuals with a wide range of prior job performance and education levels participate in a job training program, allowing for comparisons across subgroups.
- Without Overlap: The program is only offered to individuals with high prior job performance, preventing comparisons with untreated individuals with similar performance.
Checking for Overlap:
- Graphical Check: Plot the density of propensity scores for treated and untreated groups.
- Quantitative Check: Calculate the proportion of treated and untreated individuals within each stratum of propensity scores.
Stratified RCTs and Covariate Balance Checks
Stratified RCTs:
- Stratified RCTs involve dividing the population into subgroups (strata) based on covariates before randomizing the treatment within each stratum. This design ensures that treatment and control groups are balanced across covariates, facilitating a clearer isolation of treatment effects.
Covariate Balance Checks:
- Covariate Balance Checks verify that covariates are balanced between treatment and control groups after randomization, confirming the success of the randomization process. Statistical tests such as t-tests and chi-squared tests are used to assess balance.
Conclusion
These methodologies—stratified RCTs, covariate balance checks, and conditioning on propensity scores—are essential for ensuring that treatment and control groups are comparable. By applying these methods, researchers can draw valid causal inferences from both experimental and observational data, even in the absence of randomization. Understanding and implementing these concepts is crucial for advancing research and making informed decisions based on causal relationships.