Monthly Archives: July 2024
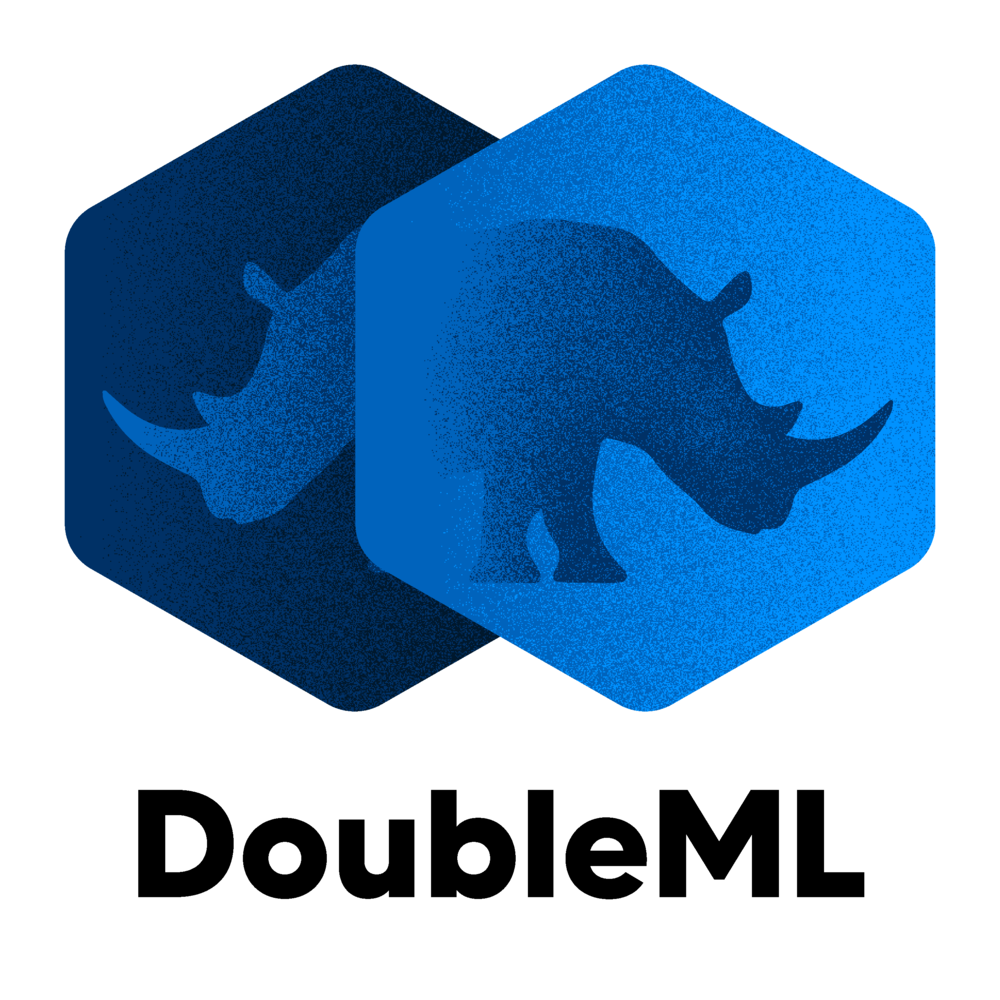
Double Machine Learning: Advancing Causal Inference with Machine Learning Techniques
Inspired by Brady Neal’s video where he humorously suggests that Double Machine Learning (DML) “is maybe twice as cool” as regular machine learning, we dive into this advanced causal framework. DML, also known as debiased machine learning or orthogonal machine learning, offers a robust method for causal inference by combining flexibility, low bias, and valid…
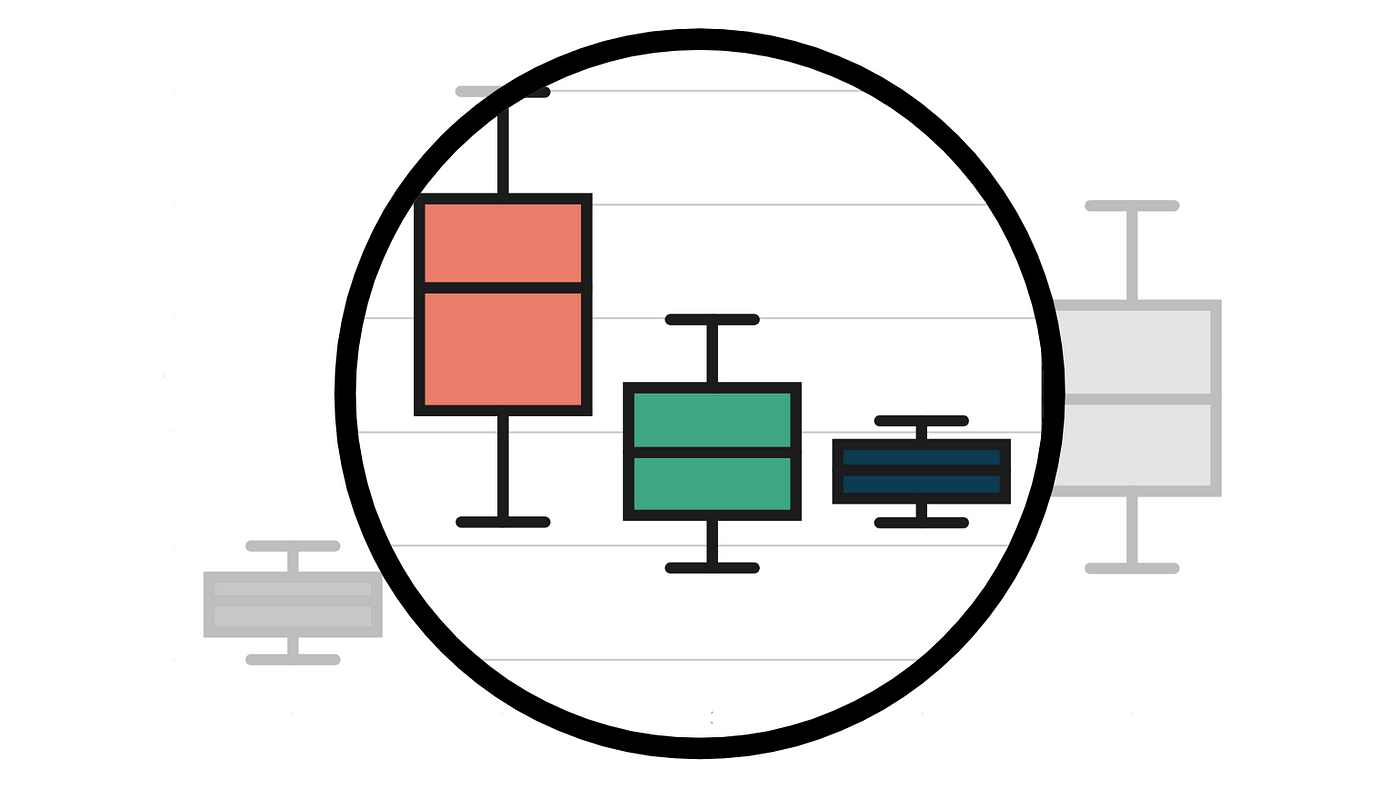
Doubly Robust Methods in Causal Inference: A Powerful Approach to Estimating Treatment Effects
In the world of causal inference, we’re constantly seeking methods that can provide accurate and reliable estimates of treatment effects. One approach that has gained significant traction in recent years is the family of Doubly Robust (DR) methods. These methods offer a unique combination of flexibility and robustness that make them particularly appealing for a…
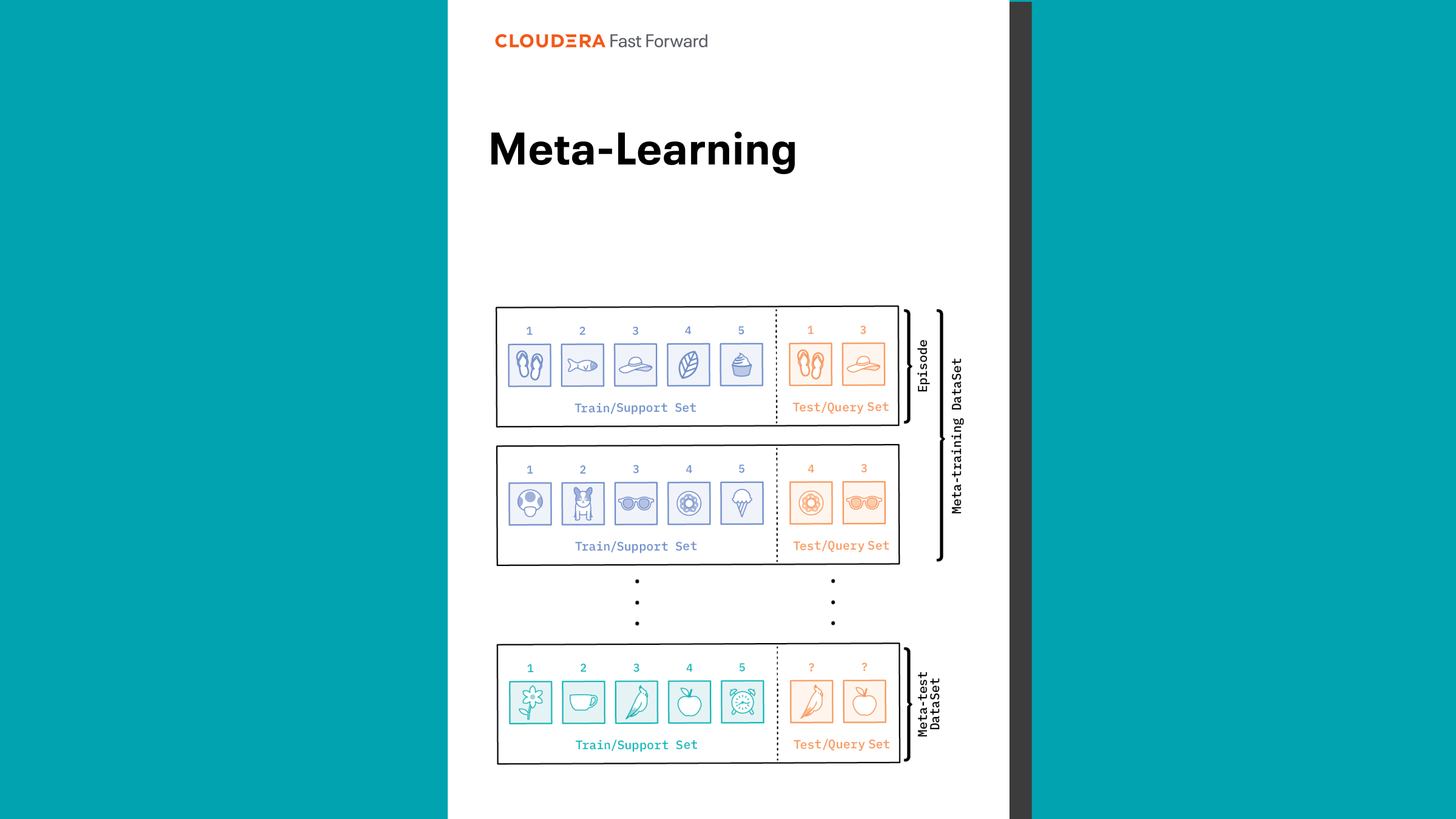
Understanding S, T, and X Learners: Meta-Learners for Causal Inference
When estimating causal effects, we often want to go beyond average treatment effects and understand how treatments impact different individuals or subgroups. This is where meta-learners like S-Learner, T-Learner, and X-Learner come in handy. Let’s explore these powerful tools for estimating heterogeneous treatment effects, with a focus on intuition and practical implementation using the DoWhy…